PS2: Uncertainty Quantification and Optimization - Response Surfaces and Machine Learning Surrogate Modelling
Monday, September 5, 2022 |
6:50 PM - 8:00 PM |
Foyer & Room 1.4 |
Speaker
Dr Emanuela Abbate
Eni Spa
Optimization Workflow Using Deep Learning Based Forward Models For Waterflooded Oil Reservoirs
6:50 PM - 6:55 PMSummary
In specific reservoir engineering problems, such as medium-term oil production forecast in waterflooded reservoirs, data-driven models are interesting alternatives to complex numerical simulations, as they can speed-up decision making without compromising accuracy.
In this work, an optimization framework using deep neural networks (DNNs) as surrogate models was established to optimize the waterflooding strategy in two synthetic cases of distinct geological complexity. Although DNNs have been tested for production forecasting in literature, the novelty of this work is the application of DNNs to optimize the injection schedule of brown fields and its comparison against a commercially available solution.
Three different families of optimization algorithms were considered: gradient-free, gradient-based, and ensemble-based. Their results are compared against a commercial simulator-based software that performs a streamline-based optimization with a given water availability target. The benchmark is run using the “true” geological model.
The first case is a 2D reservoir, with 5 injection wells and 4 production wells. It has uniform geological properties, with two high permeability streaks connecting two injector-producer pairs. The second case is Olympus, a realistic 3D reservoir with many geological heterogeneities and non-linearity sources, with 7 injection wells and 11 production wells. For each model a DNN was trained using synthetically generated historical data.
Results are compared in terms of Net Present Value (NPV) considering oil price, cost of water produced and injected, and actualization rate.
In the first case, the NPV was improved similarly by the three algorithms by reducing injections along the high permeability streaks, thus promoting the drainage of unswept areas. The benchmark achieved poor performances, promoting instead the injection in the high permeability streaks. In the second case, the three algorithms and the benchmark achieved similar NPVs with slightly different injection strategies.
The ensemble-based optimizer proved to be the best-performing algorithm, as opposed to the gradient-free which required a higher number of objective function evaluations, and the gradient-based which tended to get trapped in local optima.
The presented framework proved to be successful in optimizing the waterflooding strategy even in a complex geological setting. Compared to simulator-based optimization, the main benefit of the proposed methodology lies in its reduced computational time, both in model calibration and objective function evaluation. The time saving is especially significant when a tuned 3D model of the reservoir is unavailable or too expensive to build.
In this work, an optimization framework using deep neural networks (DNNs) as surrogate models was established to optimize the waterflooding strategy in two synthetic cases of distinct geological complexity. Although DNNs have been tested for production forecasting in literature, the novelty of this work is the application of DNNs to optimize the injection schedule of brown fields and its comparison against a commercially available solution.
Three different families of optimization algorithms were considered: gradient-free, gradient-based, and ensemble-based. Their results are compared against a commercial simulator-based software that performs a streamline-based optimization with a given water availability target. The benchmark is run using the “true” geological model.
The first case is a 2D reservoir, with 5 injection wells and 4 production wells. It has uniform geological properties, with two high permeability streaks connecting two injector-producer pairs. The second case is Olympus, a realistic 3D reservoir with many geological heterogeneities and non-linearity sources, with 7 injection wells and 11 production wells. For each model a DNN was trained using synthetically generated historical data.
Results are compared in terms of Net Present Value (NPV) considering oil price, cost of water produced and injected, and actualization rate.
In the first case, the NPV was improved similarly by the three algorithms by reducing injections along the high permeability streaks, thus promoting the drainage of unswept areas. The benchmark achieved poor performances, promoting instead the injection in the high permeability streaks. In the second case, the three algorithms and the benchmark achieved similar NPVs with slightly different injection strategies.
The ensemble-based optimizer proved to be the best-performing algorithm, as opposed to the gradient-free which required a higher number of objective function evaluations, and the gradient-based which tended to get trapped in local optima.
The presented framework proved to be successful in optimizing the waterflooding strategy even in a complex geological setting. Compared to simulator-based optimization, the main benefit of the proposed methodology lies in its reduced computational time, both in model calibration and objective function evaluation. The time saving is especially significant when a tuned 3D model of the reservoir is unavailable or too expensive to build.
Dr Hamidreza Hamdi
Research Scientist
University of Calgary
Accurate surrogate modelling for timeseries using functional principal component analysis: Application to history-matching and uncertainty quantification
6:55 PM - 7:00 PMSummary
Accurate surrogate models are essential for application of computational methods such as Markov chain Monte Carlo (McMC) using numerical reservoir simulation. Previous studies have often focused on building surrogates to represent the misfit (or likelihood) function. However, building an accurate non-negative surrogate for the likelihood is difficult for higher dimensions unless an overly large number of samples is simulated first. Fortunately, functional data analysis can provide a set of ensemble-based statistical tools which can be utilized to emulate the full simulation output rather than the misfit function itself. Consequently, the misfit can be easily calculated by the simulated timeseries.
In this study, functional principal component analysis (fPCA) is utilized to reduce the dimensionality of the timeseries. In other words, the simulation output (e.g., oil rate) is represented in terms of a few optimal functional principal component scores (fPCS), which can be readily inverted to reconstruct the original timeseries. fPCA is a favorable tool for developing a new and efficient Bayesian history matching workflow in that it can be used to iteratively update the surrogate and search for the extrema of the likelihood function. In this proposed process, a few initial random samples are generated, and the corresponding timeseries are processed by fPCA. The resulting fPCSs for each simulation output are modelled individually using kriging or Random Forests, which are then used to estimate the likelihood and optimized to suggest the next best samples until a convergence criterion is met.
This workflow is applied to a set of data obtained from a near critical gas condensate well from a Canadian shale reservoir. The proposed history matching workflow results in a 10-times faster convergence rate compared to an adaptive differential evolution algorithm. The history matching samples are combined with additional quasi-Monte Carlo samples to enhance the exploration aspect and predictability of the surrogate models. The results demonstrate that clustering of the timeseries and applying fPCA to each cluster separately can significantly enhance the accuracy of the surrogates. Finally, the surrogates are utilized to obtain accurate posteriors quickly through an McMC algorithm.
This study introduces an adaptive sampling method for the first time that can be used to generate a highly accurate surrogate for timeseries and conduct the optimization efficiently. This surrogate modelling workflow can also be used for other reservoir engineering problems such as numerically assisted rate-transient analysis where new timeseries should be predicted from a limited number of numerical simulations.
In this study, functional principal component analysis (fPCA) is utilized to reduce the dimensionality of the timeseries. In other words, the simulation output (e.g., oil rate) is represented in terms of a few optimal functional principal component scores (fPCS), which can be readily inverted to reconstruct the original timeseries. fPCA is a favorable tool for developing a new and efficient Bayesian history matching workflow in that it can be used to iteratively update the surrogate and search for the extrema of the likelihood function. In this proposed process, a few initial random samples are generated, and the corresponding timeseries are processed by fPCA. The resulting fPCSs for each simulation output are modelled individually using kriging or Random Forests, which are then used to estimate the likelihood and optimized to suggest the next best samples until a convergence criterion is met.
This workflow is applied to a set of data obtained from a near critical gas condensate well from a Canadian shale reservoir. The proposed history matching workflow results in a 10-times faster convergence rate compared to an adaptive differential evolution algorithm. The history matching samples are combined with additional quasi-Monte Carlo samples to enhance the exploration aspect and predictability of the surrogate models. The results demonstrate that clustering of the timeseries and applying fPCA to each cluster separately can significantly enhance the accuracy of the surrogates. Finally, the surrogates are utilized to obtain accurate posteriors quickly through an McMC algorithm.
This study introduces an adaptive sampling method for the first time that can be used to generate a highly accurate surrogate for timeseries and conduct the optimization efficiently. This surrogate modelling workflow can also be used for other reservoir engineering problems such as numerically assisted rate-transient analysis where new timeseries should be predicted from a limited number of numerical simulations.
Mr Junjie Yu
Research Assistant
University of Southern California
Active Learning for Efficient Optimization of Geological CO2 Storage with Surrogate Models
7:00 PM - 7:05 PMSummary
Geological CO2 storage (GCS) has been commonly recognized as an effective approach to reduce greenhouse gas emissions. The trapping mechanisms have been widely studied in the literature, where solubility and residual trapping are considered as safer for short-term entrapment. However, density-driven upward CO2 can hamper those mechanisms since the existence of free-phase CO2 would lead to the risk of CO2 leakage. One way to mitigate such risks is to adopt optimization strategies that attempt to adjust the well controls (e.g., injection rate) to maximize the immobilized CO2 (or minimize the free-phase CO2). However, model-based optimizations are computationally demanding, especially when time-consuming forward simulations such as those used for GCS are used. Surrogate models provide an attractive fit-for-purpose alternative for generating the required simulation responses at a reduced computational cost.
Recently, deep learning-based surrogate models have been proposed to speed up the optimization procedure. A major limitation of these models is their generalizability or extrapolation power, that is, their ability to predict beyond the training data, which is likely to happen during the optimization iterations. We propose an active learning strategy to address this issue by adapting the training data to the optimization iterations to improve the local accuracy of the model. Active learning is popular when unlabeled data is abundant but labeling (running simulation in our application) is expensive. One of the main advantages of active learning is that instead of frontloading the computation, it selectively and dynamically adds new data points to the training process, thereby adapting the prediction accuracy and distributing the computational budget efficiently. We apply active learning-based optimization with an artificial neural network (ANN) proxy model to maximize the immobilized CO2 during GCS. For local gradient-based optimization, active learning provides an efficient approach to adaptively sample new training data around the optimization path and update the ANN-based surrogate model to maintain its local accuracy.
Compared to the traditional off-line training approach, active learning results in improved model accuracy and computational efficiency. Active learning is a general framework that can be used in other subsurface flow applications to reduce computation and to improve the consistency between surrogate models and their corresponding full-scale simulation models.
Recently, deep learning-based surrogate models have been proposed to speed up the optimization procedure. A major limitation of these models is their generalizability or extrapolation power, that is, their ability to predict beyond the training data, which is likely to happen during the optimization iterations. We propose an active learning strategy to address this issue by adapting the training data to the optimization iterations to improve the local accuracy of the model. Active learning is popular when unlabeled data is abundant but labeling (running simulation in our application) is expensive. One of the main advantages of active learning is that instead of frontloading the computation, it selectively and dynamically adds new data points to the training process, thereby adapting the prediction accuracy and distributing the computational budget efficiently. We apply active learning-based optimization with an artificial neural network (ANN) proxy model to maximize the immobilized CO2 during GCS. For local gradient-based optimization, active learning provides an efficient approach to adaptively sample new training data around the optimization path and update the ANN-based surrogate model to maintain its local accuracy.
Compared to the traditional off-line training approach, active learning results in improved model accuracy and computational efficiency. Active learning is a general framework that can be used in other subsurface flow applications to reduce computation and to improve the consistency between surrogate models and their corresponding full-scale simulation models.
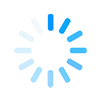