Uncertainty Quantification and Optimization - Geostatistics, Geolocical Uncertainty Modelling, Risk and Uncertainty Analysis
Tracks
Track 2
Tuesday, September 6, 2022 |
8:30 AM - 10:10 AM |
Room 1.2 |
Speaker
Dr Masoud Maleki
Research Scientist
Center for Petroleum Studies (CEPETRO)_University of Campinas
A workflow for high-resolution reservoir characterization using multiple 4D seismic datasets
8:30 AM - 8:55 AMSummary
The uncertainties and risks related to the complex environments of deepwater oil and gas reservoirs require interdisciplinary workflows and advanced reservoir monitoring techniques to surveil reservoir performance during production activities. An example of the latter is a Permanent Reservoir Monitoring (PRM) system, which provides 4D seismic data on demand. A challenge of such approach to reservoir monitoring is the rapid assimilation of frequently acquired high-quality 4D seismic data into fast-track information. Delays and limitations in the interpretation and integration of 4D seismic data reduce the benefits of such approach to impact the decision-making processes, despite obtaining data on demand. This work presents an integrated workflow of 4D seismic and reservoir engineering data to harvest hidden dynamic reservoir insights over short periods of time. This information provides novel inputs to update reservoir models to improve model-based reservoir management. Our workflow illustrates the importance of multiple 4D seismic datasets in the field management strategy. This study was carried out in a Brazilian deepwater turbidite field, where a PRM system recorded a baseline survey at the start of production in 2013 and 5 monitor surveys up to 2020. We obtain an enhancement in the interpretive capability of the dynamic reservoir behavior such as identifying regions of possible pushed oil by injectors and revealing a hidden channel of aquifer movement.
Mr Audun Sektnan
Norwegian Computing Center
A Tree Representation of Plurigaussian Truncation Rules
8:55 AM - 9:20 AMSummary
Truncated Gaussian fields are a common way of modelling facies, where the correlation structure in the Gaussian field defines a spatial correlation structure for the facies. Plurigaussian simulation takes it further by using several underlying Gaussian fields. This allows more flexibility and makes it possible to model a wider range of geological settings, but conditioning can be difficult.
We present a fast and accurate implementation of conditional plurigaussian simulation. Our approach has two key elements. The first is to combine complex truncation rules with input facies probabilities. The truncation rule, which is a function from the Gaussian fields to a facies value, can be represented neatly as a binary truncation tree. This allows for a general representation that includes all the traditional 2D truncation masks. We show how to combine the use of such trees with facies probabilities, even in complicated cases with more than two Gaussian fields.
The second key element is correct conditioning to all facies observations, not just transitions, by treating them as inequality constraints on the Gaussian fields. We perform inequality Kriging by replacing these facies observations by synthetic observations of the underlying Gaussian fields. To generate synthetic observations that agree with the target posterior distribution, we use a Gibbs sampler. Since this is a quite slow algorithm, we take certain measures to make the calculations faster. Synthetic observations are then used in Kriging, improving the conditioning to facies logs from wells. We demonstrate the method with a synthetic case that combines a large number of observations with the use of a truncation tree tailored from a geological concept.
We present a fast and accurate implementation of conditional plurigaussian simulation. Our approach has two key elements. The first is to combine complex truncation rules with input facies probabilities. The truncation rule, which is a function from the Gaussian fields to a facies value, can be represented neatly as a binary truncation tree. This allows for a general representation that includes all the traditional 2D truncation masks. We show how to combine the use of such trees with facies probabilities, even in complicated cases with more than two Gaussian fields.
The second key element is correct conditioning to all facies observations, not just transitions, by treating them as inequality constraints on the Gaussian fields. We perform inequality Kriging by replacing these facies observations by synthetic observations of the underlying Gaussian fields. To generate synthetic observations that agree with the target posterior distribution, we use a Gibbs sampler. Since this is a quite slow algorithm, we take certain measures to make the calculations faster. Synthetic observations are then used in Kriging, improving the conditioning to facies logs from wells. We demonstrate the method with a synthetic case that combines a large number of observations with the use of a truncation tree tailored from a geological concept.
Dr Emanuela Abbate
Eni Spa
Ensemble data space inversion for fast CO2 injection forecast evaluation
9:20 AM - 9:45 AMSummary
The geological storage of CO2 into depleted reservoirs represents a potential strategy for large-scale greenhouse gas mitigation. An evaluation of the storable volume and of the behaviour of the injected fluid considering the uncertainty is essential to mitigate the associated geo-mechanical risks and the potential CO2 leakage.
The accurate estimation of the quantity of CO2 that can be injected into a depleted reservoir is usually carried out after the calibration of the reservoir model. This model calibration can be addressed via a history matching process, by assimilating production and pressure data collected during the field production history. The prior uncertainties represented by an ensemble of reservoir model realizations are thus reduced, by solving a nonlinear inverse problem with computationally demanding methods such as iterative ensemble data assimilation. The history matching phase is crucial for the forecast simulation under realistic conditions of carbon capture and storage applications, but it can be time consuming especially in presence of a long historical time.
In the present work, we propose an innovative method to significantly reduce the computational impact of the calibration process, adopting a direct forecasting approach based on the ensemble data space inversion (DSI) introduced by Lima et al. [1]. With this approach, a direct prior ensemble prediction update is performed to account for the historical data, without modifying the models themselves. The Posterior (history-matched) geological models are not explicitly obtained as in standard model-space inversion methods and as consequence the requirements in term of computational resources and CPU time are strongly reduced.
The DSI approach is here implemented adopting an iterative ensemble smoother formulation tailored to quantify the uncertainty on the total volume of CO2 that can be stored into the depleted reservoir. Moreover, the method can be used to assess the uncertainty of the subsurface fluid flow. The application examples show that a calibration process via the DSI algorithm can produce accurate forecast predictions and can consistently reduce uncertainty. The results are comparable to the ones that are obtained by standard model-space inversion approaches.
[1] Lima M. M., Emerick A., and Ortiz C. EP. "Data-space inversion with ensemble smoother" Computational Geosciences 24.3 (2020): 1179-1200.
The accurate estimation of the quantity of CO2 that can be injected into a depleted reservoir is usually carried out after the calibration of the reservoir model. This model calibration can be addressed via a history matching process, by assimilating production and pressure data collected during the field production history. The prior uncertainties represented by an ensemble of reservoir model realizations are thus reduced, by solving a nonlinear inverse problem with computationally demanding methods such as iterative ensemble data assimilation. The history matching phase is crucial for the forecast simulation under realistic conditions of carbon capture and storage applications, but it can be time consuming especially in presence of a long historical time.
In the present work, we propose an innovative method to significantly reduce the computational impact of the calibration process, adopting a direct forecasting approach based on the ensemble data space inversion (DSI) introduced by Lima et al. [1]. With this approach, a direct prior ensemble prediction update is performed to account for the historical data, without modifying the models themselves. The Posterior (history-matched) geological models are not explicitly obtained as in standard model-space inversion methods and as consequence the requirements in term of computational resources and CPU time are strongly reduced.
The DSI approach is here implemented adopting an iterative ensemble smoother formulation tailored to quantify the uncertainty on the total volume of CO2 that can be stored into the depleted reservoir. Moreover, the method can be used to assess the uncertainty of the subsurface fluid flow. The application examples show that a calibration process via the DSI algorithm can produce accurate forecast predictions and can consistently reduce uncertainty. The results are comparable to the ones that are obtained by standard model-space inversion approaches.
[1] Lima M. M., Emerick A., and Ortiz C. EP. "Data-space inversion with ensemble smoother" Computational Geosciences 24.3 (2020): 1179-1200.
Dr Dimitrii Posvyanskii
Department Director
AspenTech
Well inflow modeling in reservoirs with random permeability
9:45 AM - 10:10 AMSummary
Well modeling plays a significant role in numerical reservoir simulation. A well is coupled to the reservoir through the use of well index (WI), which relates well rate and pressure of grid block. Generally , the Peaceman expression is used to calculate well index, but it was obtained under the assumption of radial well inflow in homogeneous reservoir. A real-field reservoir permeability exhibits high variability and incomplete knowledge about it allows us to treat permeability as a random function. The randomness of the permeability induces the randomness of pressure and WI. The objective of this study is to calculate low-order statistical moments of pressure and WI in reservoirs with random permeability. The variance of WI can be useful in history matching process, since it allows to estimate confidence interval for WI. The knowledge of pressure moments also allows us to use streamline approach to solve transport problem in a random reservoir and to calculate the time of flight (TOF) between producer and injector wells . Statistical characteristics of TOF can be used to assess the risks of water breakthrough.
Stochastic moment equations (SME) approach was proposed for modeling fluid flow in porous media . This equations was formulated for low-order moments of random permeability and pressure fields. The presence of wells lead to logarithmic singularities in the pressure and its variance that is difficult to resolve SME numerically . In this study SME approach is employed to describe well inflow in conjunction with singularity removal method. Explicit removal of singularities from SME allows to solve them using standard numerical methods. The approach of stochastic differential equation is used to TOF calculation. This approach was originally used to describe the movement of Brownian particle.
The calculation of WI was carried out in the case of vertical and horizontal well for given values of permeability variance and correlation length. The dependence of WI and TOF variances on the statistical characteristics of random permeability field is estimated.
Monte Carlo simulation is one of the major tools to calculate pressure and well rates in reservoirs with uncertain properties. The comparison of the present study and Monte-Carlo results demonstrates very good agreement up to log-permeability variance ~ 2 , which proves the applicability of proposed approach
to the wide range of real deposits. However, calculations within SME approach are not time-consuming as compared to Monte-Carlo simulation .
Stochastic moment equations (SME) approach was proposed for modeling fluid flow in porous media . This equations was formulated for low-order moments of random permeability and pressure fields. The presence of wells lead to logarithmic singularities in the pressure and its variance that is difficult to resolve SME numerically . In this study SME approach is employed to describe well inflow in conjunction with singularity removal method. Explicit removal of singularities from SME allows to solve them using standard numerical methods. The approach of stochastic differential equation is used to TOF calculation. This approach was originally used to describe the movement of Brownian particle.
The calculation of WI was carried out in the case of vertical and horizontal well for given values of permeability variance and correlation length. The dependence of WI and TOF variances on the statistical characteristics of random permeability field is estimated.
Monte Carlo simulation is one of the major tools to calculate pressure and well rates in reservoirs with uncertain properties. The comparison of the present study and Monte-Carlo results demonstrates very good agreement up to log-permeability variance ~ 2 , which proves the applicability of proposed approach
to the wide range of real deposits. However, calculations within SME approach are not time-consuming as compared to Monte-Carlo simulation .
Session Chair
Pallav Sarma
Chief Scientist
Tachyus
Session Co-Chair
Ahmad Sami Abushaikha
Assistant Professor
Hamad bin Khalifa University
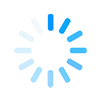