Uncertainty Quantification and Optimization - Modelling of Geological Uncertainty
Tracks
Track 2
Tuesday, September 6, 2022 |
10:40 AM - 12:20 PM |
Room 1.2 |
Speaker
Ms Tanteliniaina N. Mioratina
PhD Student
NORCE Norwegian Research Centre
Quantifying prior model complexity for subsurface reservoir models
10:40 AM - 11:05 AMSummary
In Bayesian approaches to subsurface inference, the prior model specifies the model parameters that are uncertain and the joint probability of those parameters before incorporating production-related data. A good prior model is generally complex enough to capture the future reservoir behaviour in the long term, realistic enough to be plausible, consistent with geologic knowledge, and simple enough to allow calibration for data matching. Model complexity is often associated with the number of model parameters, thus the focus on finding the sufficient number of parameters needed for history matching and to quantify uncertainty in the future.
This work explores model selection based on concepts of complexity and informativeness of models for subsurface reservoir models. It focuses on the effect of misspecification of prior models for assimilating flow data and their predictive accuracy. Using concepts of mutual information, entropy and information criteria, we investigate the suitability of various types of prior models with different level of complexity, ranging from a highly simplified bilinear trends to realistic multipoint statistical models and complex hierarchical Gaussian models and explore the effect of level of model complexity on robustness of forecasting. We perform experiments with different combinations of data type, prior informativeness, forecast type and model type to compare the effect of different prior models on robustness of the results. For each model simulation, we analyse the effective number of parameters, entropy, time required for calibration and evaluate their predictive accuracy.
We show that information content and the number of parameters are useful measures for selection of prior models for history matching. We observe that model selection according to the “widely applicable information criterion” (WAIC) gives the same results as Bayesian leave-one-out cross validation (LOO-CV) for hierarchical Gaussian priors. Experiments indicate that penalizing model complexity could be useful for models that contain parameters without physical meaning. Moreover, hierarchical Bayesian models are useful when uncertainty in prior hyper-parameters is reasonable. In addition, we suggest a workflow for model development depending on forecast objectives and data availability.
This work explores model selection based on concepts of complexity and informativeness of models for subsurface reservoir models. It focuses on the effect of misspecification of prior models for assimilating flow data and their predictive accuracy. Using concepts of mutual information, entropy and information criteria, we investigate the suitability of various types of prior models with different level of complexity, ranging from a highly simplified bilinear trends to realistic multipoint statistical models and complex hierarchical Gaussian models and explore the effect of level of model complexity on robustness of forecasting. We perform experiments with different combinations of data type, prior informativeness, forecast type and model type to compare the effect of different prior models on robustness of the results. For each model simulation, we analyse the effective number of parameters, entropy, time required for calibration and evaluate their predictive accuracy.
We show that information content and the number of parameters are useful measures for selection of prior models for history matching. We observe that model selection according to the “widely applicable information criterion” (WAIC) gives the same results as Bayesian leave-one-out cross validation (LOO-CV) for hierarchical Gaussian priors. Experiments indicate that penalizing model complexity could be useful for models that contain parameters without physical meaning. Moreover, hierarchical Bayesian models are useful when uncertainty in prior hyper-parameters is reasonable. In addition, we suggest a workflow for model development depending on forecast objectives and data availability.
Dr Daniel Busby
Senior Reservoir Engineer
TotalEnergies
3D-GAN to model uncertainty and to perform effective history matching of a complex turbidite field case
11:05 AM - 11:30 AMSummary
Modelling uncertainty of complex 3D geological fields can require several sophisticated geostatistical methods. Such methods include process like methods that mimic the physics of deposition to create more realistic geological models. Performing a full field history matching with such generative tools can be extremely challenging due to the large number of parameters that are used to generate each stochastic realization, the static data conditioning, and the complex and non-linear input-output relation. Ensemble data assimilation methods such as ESMDA (ensemble smoother with multiple data assimilation) are not directly applicable or can perform poorly due to the strong input parameter dependence and non-linearity. In this work we present some recent advances on the usage of GAN (Generative Adversarial Networks) to help solving the history matching problem when process like methods are used to generate multi-realizations to model the uncertainty of turbidite fields. GAN are deep learning generative models that are used in many AI applications. In history matching, using GAN to generate new geological models can help reducing drastically the input parameter space used to solve the inverse problem. In fact, to generate a new realization with a GAN we sample a random vector from a low dimensional independent Gaussian distribution (called the latent space). As a result, once the GAN is trained (using few thousands realizations generated with the process like methods), the inverse problem consists in having to invert only a few dozen independent parameters respect to a few millions dependent parameters in the original space.
Usage of GAN for history matching of simple synthetic field cases has been discussed in previous works. However, to apply GAN on real field cases one needs to address several issues such as:
- high number of cells of the input space (typical GAN architectures are build for 2D 128x128 images)
- highly dependent input and non-linear input output relationship
- inverse problem with multiple solutions
To obtain accurate results in our real dataset we use state-of-the-art machine learning, optimization/inversion technique and HPC. More particularly we use an advanced architecture for the 3D GAN trained on multi-GPU and a very recent Bayesian optimization technique to solve the inverse problem and to find several possible solutions. A comparison with a more industry standard approach using ESMDA is also presented and discussed.
Usage of GAN for history matching of simple synthetic field cases has been discussed in previous works. However, to apply GAN on real field cases one needs to address several issues such as:
- high number of cells of the input space (typical GAN architectures are build for 2D 128x128 images)
- highly dependent input and non-linear input output relationship
- inverse problem with multiple solutions
To obtain accurate results in our real dataset we use state-of-the-art machine learning, optimization/inversion technique and HPC. More particularly we use an advanced architecture for the 3D GAN trained on multi-GPU and a very recent Bayesian optimization technique to solve the inverse problem and to find several possible solutions. A comparison with a more industry standard approach using ESMDA is also presented and discussed.
Dr Ralf Schulze-Riegert
SLB
Well placement optimization for geothermal reservoir under subsurface uncertainty
11:30 AM - 11:55 AMSummary
As a resource for renewable energy, geothermal fields require improved methods for locating wells to maximize production. While subsurface characterization has improved, the uncertainty in derived sweet spot maps of permeability distribution is still broad. Incremental changes of predicted production due to reduced and calibrated subsurface uncertainty can have a strong impact on the economics of a project. This work investigates a multi-deterministic/multi-stochastic approach to capture uncertainties in fracture distributions of connected natural fractures that produce geothermal fluids. A practical application is demonstrated for the Darajat geothermal field, Indonesia.
Remote sensing methods, surface geology, and well data are integrated to characterize the geometry of intrusions, faults, associated pyroclastics and overlying sediments. Reservoir modelling of natural fractures is determined by “drivers,” which are built on geo-mechanical methods and fracture density variations based on distance-to-fault dependencies and cooling of the intrusion. The predictability of the geothermal reservoir permeability is determined by the validity of fracture driver contributions. Two model validation steps are introduced; first, the model prediction of observed fractures interpreted in image logs and second, the comparison of the well productivity index to the modelled permeability distribution within a volumetric neighborhood of producing wells in the field area. Multiple-objective measures are defined to automate the model validation process and to select model candidates for sweet spot analysis.
Open and effective fracture density variations across the field are critical for targeting sweet spot areas. The modelled magnitude of permeability level correlates with the measured productivity of a well. Both observations are parameterized as objective measures and are applied for model validation and selection. A probabilistic assessment including all selected model candidates is defined for robust well placement strategies. The approach couples multiple-deterministic structural scenarios and multiple-stochastic realizations of the discrete fracture network model for sweet spot analysis under subsurface uncertainties. Objective performance measures and analytics are developed for result analysis and classification.
Remote sensing methods, surface geology, and well data are integrated to characterize the geometry of intrusions, faults, associated pyroclastics and overlying sediments. Reservoir modelling of natural fractures is determined by “drivers,” which are built on geo-mechanical methods and fracture density variations based on distance-to-fault dependencies and cooling of the intrusion. The predictability of the geothermal reservoir permeability is determined by the validity of fracture driver contributions. Two model validation steps are introduced; first, the model prediction of observed fractures interpreted in image logs and second, the comparison of the well productivity index to the modelled permeability distribution within a volumetric neighborhood of producing wells in the field area. Multiple-objective measures are defined to automate the model validation process and to select model candidates for sweet spot analysis.
Open and effective fracture density variations across the field are critical for targeting sweet spot areas. The modelled magnitude of permeability level correlates with the measured productivity of a well. Both observations are parameterized as objective measures and are applied for model validation and selection. A probabilistic assessment including all selected model candidates is defined for robust well placement strategies. The approach couples multiple-deterministic structural scenarios and multiple-stochastic realizations of the discrete fracture network model for sweet spot analysis under subsurface uncertainties. Objective performance measures and analytics are developed for result analysis and classification.
Session Chair
Michael Peter Suess
Professor of Geology
Stratum Geoscience GmbH
Session Co-Chair
Alberto Cominelli
Technical Advisor
Eni S.p.A. E&P
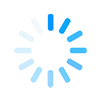