PS2: Uncertainty Quantification and Optimization - Modelling of Geological Uncertainties
Monday, September 5, 2022 |
6:50 PM - 8:00 PM |
Foyer & Room 1.2 |
Speaker
Mr Syamil Mohd Razak
University of Southern California
Adversarial Strategies for Improved Geologic Consistency in Feature-Based Parameterization
6:50 PM - 6:55 PMSummary
Forecasting fluid production from hydrocarbon reservoirs requires the construction of earth models from various sources of subsurface data. Subsequently, the observed dynamic data is integrated into the initial models to create a set of calibrated model realizations that reproduce the past dynamic data. If done correctly, the resulting calibrated models have improved predictive capability with reduced uncertainty. In practical applications, model parameters have complex (non-Gaussian) distributions, and the flow models provide a nonlinear mapping between the model responses and parameters. In these situations, classical feature-based parameterization and data assimilation techniques that rely on covariance information exhibit difficulty in capturing and preserving the complex spatial patterns.
We present new deep learning training strategies to improve the geologic consistency of feature-based parameterization techniques for ensemble data assimilation. Specifically, a neural network architecture is trained with an adversarial loss function to learn a mapping from low-rank representations to high-fidelity models, thereby improving the reconstruction quality when compared to classical dimensionality reduction techniques. The adversarial loss function estimates the probability of error between in-sample and out-of-sample models and its minimization allows the neural network architecture to learn, and thus, to generate consistent in-sample models. Additionally, to make the mapping robust against inconsistent geologic features that may be introduced by covariance-based updates, the architecture is trained to recognize out-of-sample models generated by introducing structural noise to the original in-sample models.
The proposed adversarial training strategies are designed to generate more geologically consistent features with the original training dataset. The improved consistency in the feature-based calibrated models with the adversarial loss function leads to improved production forecasts when compared to calibrated models obtained from conventional parameterization algorithms. Several numerical experiments using complex fluvial reservoirs are presented to evaluate the performance of the developed approach and compare it with other parameterization methods.
We present new deep learning training strategies to improve the geologic consistency of feature-based parameterization techniques for ensemble data assimilation. Specifically, a neural network architecture is trained with an adversarial loss function to learn a mapping from low-rank representations to high-fidelity models, thereby improving the reconstruction quality when compared to classical dimensionality reduction techniques. The adversarial loss function estimates the probability of error between in-sample and out-of-sample models and its minimization allows the neural network architecture to learn, and thus, to generate consistent in-sample models. Additionally, to make the mapping robust against inconsistent geologic features that may be introduced by covariance-based updates, the architecture is trained to recognize out-of-sample models generated by introducing structural noise to the original in-sample models.
The proposed adversarial training strategies are designed to generate more geologically consistent features with the original training dataset. The improved consistency in the feature-based calibrated models with the adversarial loss function leads to improved production forecasts when compared to calibrated models obtained from conventional parameterization algorithms. Several numerical experiments using complex fluvial reservoirs are presented to evaluate the performance of the developed approach and compare it with other parameterization methods.
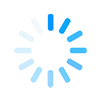