PS1: Computational Methods - Reduced Order Modelling
Monday, September 5, 2022 |
5:40 PM - 6:50 PM |
Foyer & Room 1.4 |
Speaker
Mr Maksim Elizarev
Research Assitant
Imperial College London
Efficient Dimensionality Reduction of Nonlinear Fundamental Solution for Well Placement Optimization
5:40 PM - 5:45 PMSummary
High-fidelity reservoir simulation requires substantial computational resources and time, especially when it comes to inverse problems, such as assisted history matching or well placement optimization. For acceleration purposes, a full-order model (FOM) is often approximated. Likely, the resulting proxy model has a lower spatiotemporal resolution or neglects complex physical phenomena defined by governing equations of the FOM. Ultimately, the FOM can be replaced with an artificial neural network or another pure data-driven entity.
As in other applied modeling areas such as computational fluid dynamics, one may exploit data-driven dimensionality reduction approaches to preserve governing equations within a proxy model. The concept of this approach is to collect training data from a few FOM simulations, extract patterns of this data, and then perform further simulations subject to learned patterns.
Efficient data-driven nonlinear model reduction (NMR) is often based on dimensionality reduction of a system state (e.g., pressure and saturation fields) and data-driven interpolation of nonlinear functions (e.g., PVT and mechanical properties). Combined with these two components, original equations turn into a hybrid reduced-order model (ROM), which can be solved much faster than FOM, but with similar accuracy.
Starting with Principal Component Analysis as a core tool to low-rank approximations, the variety of successful approaches to data-driven NMR is steadily growing, proposing autoencoders and other advanced techniques based on neural networks. Furthermore, the area of proxy reservoir modeling is growing itself, and there is a reasonable need for data-driven NMR approaches.
However, the mentioned inverse problems of oil recovery impose harsh requirements to the robustness of NMR algorithms. Learned from simulations with some specific distributions of field properties and well trajectories, extracted patterns should represent systems with spatial configurations beyond training data.
Thus, well placement essentially affects the location of the solution's singular points, which data-driven algorithms (in their general form) preserve by design. The corresponding patterns are inapplicable for other well locations, and dimensionality reduction becomes impractical.
This work focuses on extending the generalization ability of NMR for systems with source terms and non-uniform properties. Utilizing the apparatus of fundamental solution analysis and data-driven analysis of systems with control, we are aiming at a wider use of the advances in reliable and interpretable data-driven modeling. We propose novel approaches to variable state encoding and nonlinear fields approximation by exploiting the form of governing equations, domain-wise decompositions, and Koopman analysis.
As in other applied modeling areas such as computational fluid dynamics, one may exploit data-driven dimensionality reduction approaches to preserve governing equations within a proxy model. The concept of this approach is to collect training data from a few FOM simulations, extract patterns of this data, and then perform further simulations subject to learned patterns.
Efficient data-driven nonlinear model reduction (NMR) is often based on dimensionality reduction of a system state (e.g., pressure and saturation fields) and data-driven interpolation of nonlinear functions (e.g., PVT and mechanical properties). Combined with these two components, original equations turn into a hybrid reduced-order model (ROM), which can be solved much faster than FOM, but with similar accuracy.
Starting with Principal Component Analysis as a core tool to low-rank approximations, the variety of successful approaches to data-driven NMR is steadily growing, proposing autoencoders and other advanced techniques based on neural networks. Furthermore, the area of proxy reservoir modeling is growing itself, and there is a reasonable need for data-driven NMR approaches.
However, the mentioned inverse problems of oil recovery impose harsh requirements to the robustness of NMR algorithms. Learned from simulations with some specific distributions of field properties and well trajectories, extracted patterns should represent systems with spatial configurations beyond training data.
Thus, well placement essentially affects the location of the solution's singular points, which data-driven algorithms (in their general form) preserve by design. The corresponding patterns are inapplicable for other well locations, and dimensionality reduction becomes impractical.
This work focuses on extending the generalization ability of NMR for systems with source terms and non-uniform properties. Utilizing the apparatus of fundamental solution analysis and data-driven analysis of systems with control, we are aiming at a wider use of the advances in reliable and interpretable data-driven modeling. We propose novel approaches to variable state encoding and nonlinear fields approximation by exploiting the form of governing equations, domain-wise decompositions, and Koopman analysis.
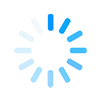