PS1: Physical Modelling - Rock Fluid Interaction and Surface Effects
Monday, September 5, 2022 |
5:40 PM - 6:50 PM |
Foyer & Room 1.4 |
Speaker
Vasyl Gnyedykh
Repsol TechLab
Manifold Learning For Realtime Log While Drilling Prediction
5:40 PM - 5:45 PMSummary
During real time drilling operations, the knowledge of rock properties and stress states while drilling are of particular importance to optimize drilling controls, assess well stability and understand reservoir characteristics. This information is often unavailable due to cost efficiency or may not cover the full depth range of interest. In this paper we propose a new mathematical framework to predict Log-While-Drilling (LWD) properties from operational parameters useful for realtime and offline evaluations. The method is particularly suited for prediction in data series with limited number of information where typical Artificial Intelligence algorithms fail in accurate prediction.
An accurate log prediction has inherent complexities due to limited data availability (number of available features), quality of data (high measurement noise) and repeatability of drilling settings (well head and perforation methods) compared to offset wells. For these reasons a new machine learning algorithm based on manifold theory has been developed. The algorithm identifies the most appropriate dataset from the online and/or offset measurements to generate a reliable model and is updated while drilling extending its coverage.
The new model has been tested to predict gamma ray logs measured while-drilling from drilling parameters in a geological complex environment. The dataset is composed by a well set with online measurement along with interpreted data. Different use cases have been considered: i) predict a missing log interval from same well measurements, ii) predict the complete log from offset wells iii) online log prediction based on offset and same well measurements.
Results show improved accuracy compared to off-the-shelf machine learning models. Particularly the algorithm has shown interesting properties that makes it differential for these types of applications: capability to capture signal changes over short intervals that are often disregarded as noise, automatic identification of analog intervals in the offset wells, fair robustness to noise and rapid re-training suitable for online and offline applications. Non less important it provides explainability to the results and evidence for the uncertainty in prediction. Even though tested on gamma ray the algorithm is well suited for all type of log prediction.
At author knowledge this is the first application of manifold analysis applied to well log data providing superior performance compared to off-the-shelf machine learning models. The methodology has been implemented in a real tool to support drilling operations and provides a step forward in drilling operation optimization.
An accurate log prediction has inherent complexities due to limited data availability (number of available features), quality of data (high measurement noise) and repeatability of drilling settings (well head and perforation methods) compared to offset wells. For these reasons a new machine learning algorithm based on manifold theory has been developed. The algorithm identifies the most appropriate dataset from the online and/or offset measurements to generate a reliable model and is updated while drilling extending its coverage.
The new model has been tested to predict gamma ray logs measured while-drilling from drilling parameters in a geological complex environment. The dataset is composed by a well set with online measurement along with interpreted data. Different use cases have been considered: i) predict a missing log interval from same well measurements, ii) predict the complete log from offset wells iii) online log prediction based on offset and same well measurements.
Results show improved accuracy compared to off-the-shelf machine learning models. Particularly the algorithm has shown interesting properties that makes it differential for these types of applications: capability to capture signal changes over short intervals that are often disregarded as noise, automatic identification of analog intervals in the offset wells, fair robustness to noise and rapid re-training suitable for online and offline applications. Non less important it provides explainability to the results and evidence for the uncertainty in prediction. Even though tested on gamma ray the algorithm is well suited for all type of log prediction.
At author knowledge this is the first application of manifold analysis applied to well log data providing superior performance compared to off-the-shelf machine learning models. The methodology has been implemented in a real tool to support drilling operations and provides a step forward in drilling operation optimization.
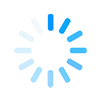