PS2: Uncertainty Quantification and Optimization
Monday, September 5, 2022 |
6:50 PM - 8:00 PM |
Foyer & Room 1.2 |
Speaker
Mr Gleb Shishaev
Engineer
Tomsk Polytechic University
History Matching And Uncertainty Quantification Of Reservoir Performance With Generative Deep Learning And Graph Convolutions
6:50 PM - 6:55 PMSummary
Generative deep learning is becoming a widely used approach in geological modelling, especially in problems that involve optimization processes under uncertainty like history matching. The basic idea of reservoir modelling and history matching by generative deep learning is to train some kind of reservoir generator and provide optimization under different constraints. There are different approaches that have been developed to utilize various modifications of Generative Adversarial Networks or Variational Autoencoders to implement reservoir generators, but most of them have “conventional” convolution neural networks, hence all data have to be regular (rectangular) in structure. This should be recognized as a limitation.
In this work, we introduce a novel approach of reservoir modelling with Variational Autoencoders based on graph convolutions as opposed to “conventional” convolutions. In our approach reservoir model is considered as a graph, i.e., not a structured data type. Graph convolutions can deal with these data types, and connection with Variational Autoencoders provides us with the capability to generate initially unstructured reservoirs. Variational Autoencoders (VAE) demonstrate the ability to implicitly parameterize geological representations into a latent space of reduced dimensionality and provide ways to uncertainty quantification and production profiling among various geological concepts.
In the first part, we introduce the motivation to Graph Variational Autoencoders (GVAE) as opposed to conventional Generative Deep learning models. The rest of the paper is dedicated to experiments on a synthetic dataset with two different geological scenarios. We show that trained GVAE performs the generation of reservoir models with reliable geology. A latent space structure between Encoder and Decoder of GVAE is represented, and interpretation considering geology under investigation is performed. Finally, we show how latent space can help to estimate the uncertainty of production and an optimization workflow under well data constraints.
In this work, we introduce a novel approach of reservoir modelling with Variational Autoencoders based on graph convolutions as opposed to “conventional” convolutions. In our approach reservoir model is considered as a graph, i.e., not a structured data type. Graph convolutions can deal with these data types, and connection with Variational Autoencoders provides us with the capability to generate initially unstructured reservoirs. Variational Autoencoders (VAE) demonstrate the ability to implicitly parameterize geological representations into a latent space of reduced dimensionality and provide ways to uncertainty quantification and production profiling among various geological concepts.
In the first part, we introduce the motivation to Graph Variational Autoencoders (GVAE) as opposed to conventional Generative Deep learning models. The rest of the paper is dedicated to experiments on a synthetic dataset with two different geological scenarios. We show that trained GVAE performs the generation of reservoir models with reliable geology. A latent space structure between Encoder and Decoder of GVAE is represented, and interpretation considering geology under investigation is performed. Finally, we show how latent space can help to estimate the uncertainty of production and an optimization workflow under well data constraints.
Dr Yuqing Chang
Equinor
An Ensemble-Based Decision-Making Workflow for Reservoir Management
6:55 PM - 7:00 PMSummary
It is challenging to make optimal field development and reservoir management decisions with diminishing resources and low-emission requirements. Thus, it is necessary to introduce advanced digital tools and account for geological uncertainty in the decision-making process. This paper demonstrates an ensemble-based probabilistic decision-making workflow for closed-loop reservoir management.
The workflow uses advanced ensemble-based history matching methods to update a reservoir model as part of the annual maturation process. The workflow is demonstrated on a field case provided by the oil&gas industry. The field case is provided with an ensemble of geological models to represent the geological uncertainty. We take the study case as a greenfield and perform optimization to decide the drilling priorities of two new wells. The new wells are open for production right after the drilling completion. We then collect the production data from existing wells and use Subspace ensemble randomized maximum likelihood (EnRML) for the history matching process. Based on the updated geological models, we optimize the drilling priorities and decide to drill new wells again. This process is repeated iteratively during the reservoir’s lifetime. As more wells are drilled and more production data are acquired during the reservoir lifetime, we learned about the reservoir properties through history matching, such as permeability, porosity and the existing fault transmissibilities. The study also shows that history matching helps to update the geological models and better understand model uncertainty, which can assist the optimization step in obtaining more robust solutions.
The approach allows for robust and up-to-date predictions, including uncertainty estimates, leading to improved decision support for field development, well-planning, production strategies, and reservoir management. We show how ensemble methods allow integrated systems for uncertainty prediction, history matching and robust optimization.
The workflow uses advanced ensemble-based history matching methods to update a reservoir model as part of the annual maturation process. The workflow is demonstrated on a field case provided by the oil&gas industry. The field case is provided with an ensemble of geological models to represent the geological uncertainty. We take the study case as a greenfield and perform optimization to decide the drilling priorities of two new wells. The new wells are open for production right after the drilling completion. We then collect the production data from existing wells and use Subspace ensemble randomized maximum likelihood (EnRML) for the history matching process. Based on the updated geological models, we optimize the drilling priorities and decide to drill new wells again. This process is repeated iteratively during the reservoir’s lifetime. As more wells are drilled and more production data are acquired during the reservoir lifetime, we learned about the reservoir properties through history matching, such as permeability, porosity and the existing fault transmissibilities. The study also shows that history matching helps to update the geological models and better understand model uncertainty, which can assist the optimization step in obtaining more robust solutions.
The approach allows for robust and up-to-date predictions, including uncertainty estimates, leading to improved decision support for field development, well-planning, production strategies, and reservoir management. We show how ensemble methods allow integrated systems for uncertainty prediction, history matching and robust optimization.
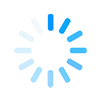