Uncertainty Quantification and Optimization - Response surfaces and Machine learning.
Tracks
Track 1
Tuesday, September 6, 2022 |
1:20 PM - 3:00 PM |
Room 1.1 |
Speaker
Mr Vinicius Silva
Imperial College London
Rapid modelling of reactive transport in porous media using machine learning
1:20 PM - 1:45 PMSummary
Reactive transport in porous media can play an important role in a variety of processes in subsurface reservoirs, such as groundwater flow, geothermal heat production, oil recovery and CO2 storage. However, numerical solution of fluid flow in porous media coupled with chemical reaction is very computationally demanding. Simultaneously, the success of machine learning in different fields has opened up new possibilities in reactive transport simulations. In this project, we focus on using machine learning techniques to replace the geochemical kinetic calculations generated by PHREEQC. PHREEQC is an open-source aqueous geochemical code that can be used in stand-alone mode or as a reaction module coupled with a flow and transport simulator. Here, we apply machine learning approaches to produce a fast proxy model of PHREEQC. This enables us to have a coupling between transport and reaction while minimizing the added computational cost. We focus initially on calcite dissolution during CO2 sequestration. Different machine learning techniques are investigated and compared to see which is more appropriate for the calcite dissolution problem. The proposed machine learning approach is designed to deal with different time-step sizes and unstructured elements. It accelerates the numerical simulation and proves to be practical to replace the reaction model presented in PHREEQC. This considerably reduces the computational cost of reactive transport while ensuring excellent simulation accuracy. The rapid modelling of reactive transport in porous media has a broad potential to replace many other phase equilibrium models across a wide range of reactive transport problems.
Prof. Ahmed Elsheikh
Professor
Heriot-Watt University
Robust well-production control using surrogate assisted reinforcement learning
1:45 PM - 2:10 PMSummary
Reinforcement Learning (RL) algorithms have been shown to achieve superhuman performance on several challenging computer games (e.g., Chess, GO). More recently, RL algorithm were utilized to solve various control problems in applied engineering fields including manufacturing, renewable energy generation and fluid dynamics drag control. These algorithms essentially learn a deep neural network (aka. policy network) that maps the system states or observations to the optimal actions or controls. In contrast, standard frameworks for solving nonlinear control problems utilize a two-stage approach. The first is a model calibration step where all the available observations are used to fit the model. The second is an optimization step where predictions obtained from the calibrated model are used to find optimal actions. This two-step approach relies on repeated model calibration whenever a new set of observations are available. As a result, it is vulnerable to overfitting where the calibrated model underestimates the uncertainties in the model parameters.
RL algorithms side-step the model calibration phase and learns optimal control policies by repeated interactions with the un-calibrated flow models. This is conceptually similar to exploration-exploitation based optimization algorithms and requires a large number of flow simulations. In this work, we utilize a combination of low fidelity proxy-models with the high-fidelity simulations to reduce the computational cost of RL algorithms. Intuitively, proxy models are utilized for the initial sampling (exploration phase) of the RL algorithms. Following that, the control policies are refined using high fidelity simulations resulting in significant computational gains. The combined use of proxy models and full-scale simulations is effectively formulated as a multi-fidelity RL framework for optimal control of physical systems governed by a set of partial differential equations (PDEs). A novel technique based on domain randomization and clustering is developed to account for the model parameters uncertainties (e.g., subsurface properties like the permeability field).
The proposed framework is demonstrated using a state-of-the-art, model-free policy based RL algorithm called proximal policy optimisation (PPO) on two subsurface flow test cases representing two distinct uncertainty distributions of the permeability field. The results are benchmarked against optimisation results obtained using differential evolution (DE) algorithm. The robustness of the learned control policy is demonstrated on unseen model parameter samples that were not used during the training process. In terms of computational efficiency, we observe significant saving in simulation runtime (approx. 60 to 70%) when utilizing the proposed multi-fidelity RL framework when compared to RL with high fidelity simulations.
RL algorithms side-step the model calibration phase and learns optimal control policies by repeated interactions with the un-calibrated flow models. This is conceptually similar to exploration-exploitation based optimization algorithms and requires a large number of flow simulations. In this work, we utilize a combination of low fidelity proxy-models with the high-fidelity simulations to reduce the computational cost of RL algorithms. Intuitively, proxy models are utilized for the initial sampling (exploration phase) of the RL algorithms. Following that, the control policies are refined using high fidelity simulations resulting in significant computational gains. The combined use of proxy models and full-scale simulations is effectively formulated as a multi-fidelity RL framework for optimal control of physical systems governed by a set of partial differential equations (PDEs). A novel technique based on domain randomization and clustering is developed to account for the model parameters uncertainties (e.g., subsurface properties like the permeability field).
The proposed framework is demonstrated using a state-of-the-art, model-free policy based RL algorithm called proximal policy optimisation (PPO) on two subsurface flow test cases representing two distinct uncertainty distributions of the permeability field. The results are benchmarked against optimisation results obtained using differential evolution (DE) algorithm. The robustness of the learned control policy is demonstrated on unseen model parameter samples that were not used during the training process. In terms of computational efficiency, we observe significant saving in simulation runtime (approx. 60 to 70%) when utilizing the proposed multi-fidelity RL framework when compared to RL with high fidelity simulations.
Dr Jeroen Vink
Shell Global Solutions Intl. B.V.
A Machine-Learning-Accelerated Distributed LBFGS Method for Field Development Optimization: Algorithm, Validation, and Applications
2:35 PM - 3:00 PMSummary
We have developed a support vector regression (SVR) accelerated variant of the distributed derivative-free optimization (DFO) method using the limited-memory BFGS Hessian updating formulation (LBFGS) for subsurface field-development optimization problems. The SVR enhanced distributed LBFGS (D-LBFGS) optimizer is designed to effectively locate multiple local optima of highly nonlinear optimization problems subject to numerical noise. It operates both on single- and multiple-objective field-development optimization problems.
The basic D-LBFGS DFO optimizer runs multiple optimization threads in parallel and uses the linear interpolation method to approximate the sensitivity matrix of simulated responses with respect to optimized model parameters. However, this approach is less accurate and slows down convergence. In this paper, we implement an effective variant of the SVR method, namely ε-SVR, and integrate it into the D-LBFGS engine in synchronous mode within the framework of a versatile optimization library inside a next-generation reservoir simulation platform. Because ε-SVR has a closed-form of predictive formulation, we analytically calculate the approximated objective function and its gradients with respect to input model variables subject to optimization. We investigate two different methods to propose a new search point for each optimization thread in each iteration through seamless integration of SVR with the D-LBFGS optimizer. The first method estimates the sensitivity matrix and the gradients directly using the analytical ε-SVR surrogate and then solves a LBFGS trust-region subproblem (TRS). The second method applies a trust-region search LBFGS method to optimize the approximated objective function using the analytical ε-SVR surrogate within a box-shaped trust region.
We first show that ε-SVR provides accurate estimates of gradient vectors on a set of nonlinear analytical test problems. We then report the results of numerical experiments conducted using the newly proposed SVR-enhanced D-LBFGS algorithms on both synthetic and realistic field-development optimization problems. We demonstrate that these algorithms operate effectively on realistic nonlinear optimization problems subject to numerical noise. We show that both SVR-enhanced D-LBFGS variants converge faster and thereby provide a significant acceleration over the basic implementation of D-LBFGS with linear interpolation.
The basic D-LBFGS DFO optimizer runs multiple optimization threads in parallel and uses the linear interpolation method to approximate the sensitivity matrix of simulated responses with respect to optimized model parameters. However, this approach is less accurate and slows down convergence. In this paper, we implement an effective variant of the SVR method, namely ε-SVR, and integrate it into the D-LBFGS engine in synchronous mode within the framework of a versatile optimization library inside a next-generation reservoir simulation platform. Because ε-SVR has a closed-form of predictive formulation, we analytically calculate the approximated objective function and its gradients with respect to input model variables subject to optimization. We investigate two different methods to propose a new search point for each optimization thread in each iteration through seamless integration of SVR with the D-LBFGS optimizer. The first method estimates the sensitivity matrix and the gradients directly using the analytical ε-SVR surrogate and then solves a LBFGS trust-region subproblem (TRS). The second method applies a trust-region search LBFGS method to optimize the approximated objective function using the analytical ε-SVR surrogate within a box-shaped trust region.
We first show that ε-SVR provides accurate estimates of gradient vectors on a set of nonlinear analytical test problems. We then report the results of numerical experiments conducted using the newly proposed SVR-enhanced D-LBFGS algorithms on both synthetic and realistic field-development optimization problems. We demonstrate that these algorithms operate effectively on realistic nonlinear optimization problems subject to numerical noise. We show that both SVR-enhanced D-LBFGS variants converge faster and thereby provide a significant acceleration over the basic implementation of D-LBFGS with linear interpolation.
Session Chair
Ralf Schulze-Riegert
SLB
Session Co-Chair
Daniel Busby
Senior Reservoir Engineer
TotalEnergies
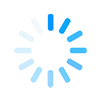