PS2: Uncertainty Quantification and Optimization - Optimization and Planning
Monday, September 5, 2022 |
6:50 PM - 8:00 PM |
Foyer & Room 1.4 |
Speaker
Mr Esmail Eltahan
University Of Texas At Austin
A quasi-Newton trust-region method for optimization under uncertainty using stochastic simplex approximate gradients
6:50 PM - 6:55 PMSummary
The goal of field-development optimization is maximizing the expected value of an objective function, e.g., net present value for a producing oil field or amount of CO2 stored in a subsurface formation, over an ensemble of models that describe the uncertainty range. A single evaluation of the objective function requires solving a system of partial differential equations, which can be computationally costly. Hence, it is most desirable for an optimization algorithm to reduce the number of objective-function evaluations while delivering high convergence rate. Here, we develop a quasi-Newton method that builds on approximate evaluations of objective-function gradients and takes more effective iterative steps using a trust-region approach compared to line search.
We implement three gradient formulations: ensemble optimization (EnOpt) gradient, and two variants of the stochastic simplex approximate gradient (StoSAG), all computed using perturbations around the point of interest. We modify the formulations to enable exploiting the objective-function structure. Instead of returning a single value for the gradient, the reformulation breaks up the objective function into its sub-components and returns a set of sub-gradients. We then can utilize our prior problem-specific knowledge through passing a ‘weight’ matrix to act on the sub-gradients. Two quasi-Newton updating algorithms are implemented: Broyden–Fletcher–Goldfarb–Shanno and the symmetric rank 1. We first evaluate the variants of our method on test challenging functions (e.g., stochastic variants of Rosenbrock and Chebyquad). Then, we present an application of well-control optimization for a realistic synthetic case.
Our results confirm that StoSAG gradients are significantly more effective than EnOpt gradients for accelerating convergence. An important challenge to stochastic gradients is determining a-priori the adequate number of perturbations. We report that the optimal number of perturbations depends on both the number of decision variables and the size of uncertainty ensemble and provide practical guidelines for its selection. We show on the test functions that imposing our prior knowledge on the problem structure can improve the gradient quality and significantly accelerate convergence. In many instances, the quasi-Newton algorithms deliver superior performance compared to the steepest-descent algorithm, especially during the early iterations. Given the computational cost involved in typical applications, rapid and noteworthy improvements at early iterations is greatly desirable for accelerated project delivery. Furthermore, our method is robust, exploits parallel processing, and can be readily applied in a generic fashion for a variety of problems where the true gradient is difficult to compute or simply not available.
We implement three gradient formulations: ensemble optimization (EnOpt) gradient, and two variants of the stochastic simplex approximate gradient (StoSAG), all computed using perturbations around the point of interest. We modify the formulations to enable exploiting the objective-function structure. Instead of returning a single value for the gradient, the reformulation breaks up the objective function into its sub-components and returns a set of sub-gradients. We then can utilize our prior problem-specific knowledge through passing a ‘weight’ matrix to act on the sub-gradients. Two quasi-Newton updating algorithms are implemented: Broyden–Fletcher–Goldfarb–Shanno and the symmetric rank 1. We first evaluate the variants of our method on test challenging functions (e.g., stochastic variants of Rosenbrock and Chebyquad). Then, we present an application of well-control optimization for a realistic synthetic case.
Our results confirm that StoSAG gradients are significantly more effective than EnOpt gradients for accelerating convergence. An important challenge to stochastic gradients is determining a-priori the adequate number of perturbations. We report that the optimal number of perturbations depends on both the number of decision variables and the size of uncertainty ensemble and provide practical guidelines for its selection. We show on the test functions that imposing our prior knowledge on the problem structure can improve the gradient quality and significantly accelerate convergence. In many instances, the quasi-Newton algorithms deliver superior performance compared to the steepest-descent algorithm, especially during the early iterations. Given the computational cost involved in typical applications, rapid and noteworthy improvements at early iterations is greatly desirable for accelerated project delivery. Furthermore, our method is robust, exploits parallel processing, and can be readily applied in a generic fashion for a variety of problems where the true gradient is difficult to compute or simply not available.
Mr Ayzat Latipov
V. A. Trapeznikov Institute Of Control Sciences Of Russian Academy Of Sciences
Optimal placement of production wells on a gas field with uniform distribution of reserves between wells
6:55 PM - 7:00 PMSummary
We propose a modification of an optimal producer wells placement model on a gas reservoir. The basic formulation of the problem is as follows: the gas reservoir is approximated by a two-dimensional map consisting of identical square blocks, each of which may or may not contain a well bottom. We need to find the blocks in which the defined number of producers will be placed optimizing certain criteria, namely, maximum approach of wells to areas with the largest reserves, and maximum coverage of the reservoir by wells. Previously, the solution was to divide the reservoir into zones of influence, similar to drainage areas. Each zone consisted of the same number of blocks. The advance of the new model is the introduction of conditions: an avoidance of gaps occurrences in zones of wells’ influence, and the uniform distribution of gas reserves among wells. Thus, we avoid the cases of having "alien" blocks in a zone belonging to other zones of other wells’ influence; furthermore, the total gas reserves are equally divided between all the wells. At the same time, due to uneven geological distribution of reserves over the field area, the number of blocks in zones of wells’ influence may vary. An admissible degree of violation of the condition of uniform distribution of reserves among the wells is introduced. Described changes cause changes to the solution algorithm. A bi-criterial discrete programming problem to be solved: one criterion is to minimize the total penalty for violation of rational well placement conditions, and another one is to minimize the number of "alien" blocks in the zones of wells’ influence.
In contrast to different optimal well placement algorithms, the proposed algorithms yield irregular well patterns. Thus, algorithms tend to find the most preferable location of an individual well considering the location of other wells. Therefore, the proposed model of optimal well placement is more consistent with the practice and peculiarities of natural gas field development. The purpose of this work was to increase the degree of adequacy of optimal well placement models to real geological and production conditions of natural gas fields development.
We present examples of problem solutions with different condition violation degree values of uniform reserves distribution between producers.
In contrast to different optimal well placement algorithms, the proposed algorithms yield irregular well patterns. Thus, algorithms tend to find the most preferable location of an individual well considering the location of other wells. Therefore, the proposed model of optimal well placement is more consistent with the practice and peculiarities of natural gas field development. The purpose of this work was to increase the degree of adequacy of optimal well placement models to real geological and production conditions of natural gas fields development.
We present examples of problem solutions with different condition violation degree values of uniform reserves distribution between producers.
Prof. Dr Mustafa Onur
McMan Chair Professor and Chairman
The University of Tulsa,
Multi-objective Optimization Of Subsurface CO2 Capture And Storage Using Sequential Quadratic Programming With Stochastic Gradients
7:00 PM - 7:05 PMSummary
Carbon capture, utilization, and storage (CCUS) is a crucial part of the energy industry nowadays, aiming to reduce the overall carbon emission into the environment. One solution to CCUS is via the means of CO2 enhanced recovery processes in a depleted oil reservoir. In such a case, life-cycle production optimization plays a crucial component, referring to optimizing a production-driven objective function via varying well controls during a reservoir's lifetime. One challenge is to obtain the optimal cash flow while trying to maintain the maximum CO2 storage. Another challenge is the nonlinear constraints (such as field liquid production rate) which need to be honored due to the capacity of the processing facilities. This study presents an application of a stochastic gradient-based framework to solve the CO2 storage multi-objective optimization problem. Our study focuses on carbon capture and storage via the means of nonlinearly constrained production optimization workflow for a CO2 enhanced recovery process, in which we aim to bi-objectively maximize both the net-present-value (NPV) and the net present carbon tax credits (NPCTC). The main framework used in this work is line-search sequential quadratic programming (LS-SQP) with stochastic simplex approximated gradients (StoSAG). We demonstrate the performance and results of the algorithmic framework in a field-scale realistic problem. The case study being investigated is a multiphase flow Brugge model under CO2 injection, simulated using a commercial compositional reservoir simulator. Results show that the LS-SQP algorithm with StoSAG gradients performs computationally efficiently and effectively in handling nonlinear state constraints imposed onto the problem. The workflow successfully solves both the single-objective and the multi-objective optimization problems with minimal and acceptable constraint violations. Various numerical settings have been experimented with to estimate the Pareto front for the bi-objective optimization problem, showing the trade-off between the two objectives NPV and NPCTC. We have demonstrated an approach to the carbon capture, utilization, and storage (CCUS) in the context of multi-objective production optimization of a CO2 enhanced recovery process for a field-scale realistic reservoir model. The algorithmic framework used in this study has proven to be computationally effective on the problem and especially useful when utilized in conjunction with commercial flow simulators that lack the capability of computing adjoint-based gradients.
Dr Cong Xiao
China University Of Petroleum, Beijing
Hierarchical surrogate-assisted evolutionary algorithm with variable-reduction strategy for well control optimization
7:05 PM - 7:10 PMSummary
Maximizing the net present value (NPV) in the process of reservoir management through recognizing optimal well-controlling conditions rapidly is critical to enhance the hydrocarbon recovery in the petroleum industry. Traditionally, this task was performed by testing various design scenarios through repeatedly running highly complicated physical models and therefore presents computational intractability and infeasibility in mathematical optimization. Therefore, we propose a computation-efficient and intelligent optimization framework, which considerably reduces computation-burden by exploiting state-of-the-art multi-fidelity modeling technology. Specifically, the low-fidelity radial-basis function (RBF) surrogate is used to prescreen the individuals globally, while the high-fidelity physic models are employed to yield the next generations. Beside, two type of multiscale variable-reduction strategies, i.e., function control method (FCM) and interpolation control method (ICM), are employed to regularize well-controls to improve convergence performance in the process of evolutionary optimization. We further introduce a transformation function to make the well control constrains automatically satisfied and therefore ease the implementation of entire optimization framework. The performance of the new approach has been initially assessed through four benchmark test functions, and then further applied to a synthetic 2D channelized model and a benchmark 3D reservoir model for practical production optimization demonstration. The obtained results show that our proposed surrogate-based optimization approach is significantly efficient to obtain satisfactory results. The use of variable reduction is capable of searching the optimal solution in a highly compressed dimension space and therefore prompting the optimization process to reach convergence rapidly, which allows much fewer full-physic simulations to significantly improve the NPV values within a very short time frame. Both the FCM and ICM produce optimal solutions with smooth well control profile, which will benefit the realistic operation due to the easy adjustment of smooth well controls. The interpolation control method (ICM) demonstrates better performance than that of function control method (FCM), especially for the realistic production optimization cases. Increasing the dimension of reduced variables generally result in a higher NPV, along with a slower convergence and more simulation runs. There is a trade-off between computation speedup and solution quality. This hybrid workflow can be easily extended to other engineering applications related to intensive simulation-based optimization. Some other tasks, such as joint optimization or multi-objective optimization in reservoir management and development also can benefit from our proposed optimization framework.
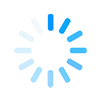