PS1: Computational Methods - Upscaling and Multiscale Methods
Monday, September 5, 2022 |
5:40 PM - 6:50 PM |
Foyer & Room 1.4 |
Speaker
Dr Mayur Pal
Professor Dept. Of Mathematical Modelling, KTU, Lithuania
Kaunas University of Technology
The Neural Upscaling Method for Single-Phase flow in Porous Medium
5:40 PM - 5:45 PMSummary
A neural upscaling methodology is introduced for upscaling heterogeneous permeability field in porous media. Traditionally, permeability upscaling is carried out either using analytical upscaling methods like arithmetic/harmonic averaging or numerical upscaling methods like local/global flow-based averaging. Analytical upscaling methods are only accurate for layered or homogeneous medium. Numerical upscaling methods have higher accuracy on heterogeneous media but involve large computational cost and results are dependent on choice of boundary conditions. Neither of the two methods account for uncertainty in geological heterogeneity. Accuracy of these methods have only been demonstrated for engineered or known permeability distribution. The Neural upscaling method proposed in this paper is built upon the framework of deep learning from large number of geological realizations, which accounts for uncertainty in geology. The Neural upscaling method is not bounded by choice of boundary conditions. Neural upscaling method is based on deep machine learning algorithms involving a multilayer neural network and could serve as a more effective alternative to standard upscaling methods, which is also computationally fast and accurate. Series of 2D test cases, where upscaling is carried out using neural networks, are presented in this paper to demonstrate the accuracy of the method. Comparisons of the neural upscaling method with numerical and analytical upscaling are also presented.
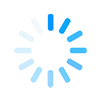