Computational Methods - ML and Data-driven/Hybrid Methods I
Tracks
Track 1
Tuesday, September 6, 2022 |
8:30 AM - 10:10 AM |
Room 1.1 |
Speaker
Mr Pijus Makauskas
Student
KTU
Neural solution to elliptic PDE with discontinuous coefficients for flow in porous media
8:30 AM - 8:55 AMSummary
Locally conservative finite-volume schemes have been developed for solving the general tensor pressure equation of petroleum reservoir-simulation on structured and unstructured grids. The schemes are applicable to diagonal and full tensor pressure equation with generally discontinuous coefficients and remove the O (1) errors introduced by standard reservoir simulation schemes when applied to full tensor flow approximation.
Two-point flux schemes (TPFA) are not applicable to full-tensor permeability and multi-Point flux approximation schemes (MPFA) has a major drawback that when it is applied to strongly anisotropic heterogeneous media as it fails to satisfy a maximum principle and result in loss of solution monotonicity for high anisotropy ratios causing spurious oscillations in the numerical pressure solution. Although variations of TPFA and MPFA have been proposed, challenges related to application on highly heterogeneous and anisotropic media still exists.
In this paper a Neural solution method to general tensor elliptic PDE with discontinuous coefficients is presented. The Neural solution to elliptic PDE is based on utilization of a deep learning multi-layer neural network, which could serve as a more effective alternative to TPFA or MPFA type schemes for fast and accurate results. Series of 2D test cases are presented, where the results of Neural solutions are compared with numerical solution using TPFA and MPFA schemes with range of heterogeneities demonstrating general applicability and accuracy of the Neural solution method. Order of accuracy of the method is compared with the numerical solution using a measure of error like the L2 norm, which shows convergence with refinement study. Neural solution for specific cases is also tested on a range of general grid types.
KEYWORDS: Porous-media, permeability tensor, MPFA, TPFA, Neural Network (NN), gridding, errors, and deep learning.
Two-point flux schemes (TPFA) are not applicable to full-tensor permeability and multi-Point flux approximation schemes (MPFA) has a major drawback that when it is applied to strongly anisotropic heterogeneous media as it fails to satisfy a maximum principle and result in loss of solution monotonicity for high anisotropy ratios causing spurious oscillations in the numerical pressure solution. Although variations of TPFA and MPFA have been proposed, challenges related to application on highly heterogeneous and anisotropic media still exists.
In this paper a Neural solution method to general tensor elliptic PDE with discontinuous coefficients is presented. The Neural solution to elliptic PDE is based on utilization of a deep learning multi-layer neural network, which could serve as a more effective alternative to TPFA or MPFA type schemes for fast and accurate results. Series of 2D test cases are presented, where the results of Neural solutions are compared with numerical solution using TPFA and MPFA schemes with range of heterogeneities demonstrating general applicability and accuracy of the Neural solution method. Order of accuracy of the method is compared with the numerical solution using a measure of error like the L2 norm, which shows convergence with refinement study. Neural solution for specific cases is also tested on a range of general grid types.
KEYWORDS: Porous-media, permeability tensor, MPFA, TPFA, Neural Network (NN), gridding, errors, and deep learning.
Dr Olwijn Leeuwenburgh
TNO
Application of coupled flow network and machine learning models for data-driven forecasting of reservoir souring
8:55 AM - 9:20 AMSummary
Flow networks, in which flow paths are approximated by one-dimensional flow tubes, have recently appeared as a potentially powerful data-physics subsurface reservoir surrogate modelling technique. Such models are fast, because the number of grid cells is considerably reduced when compared to conventional numerical reservoir model grids. Flow network models can be generated and trained directly from data rather than constructed through reduction of high-fidelity models and can be simulated using existing industry-standard reservoir simulators. The first objective of this paper is to summarize recent extensions of an open-source framework for creating and training flow networks, called FlowNet, and to demonstrate its application to a complex oil field case.
Despite their flexibility, the application of flow networks will be limited to physics that can be simulated with the available simulators. Prediction of phenomena with poorly understood underlying physics, chemistry, or, as in the case of reservoir souring, microbial ecology, may require a more data-driven approach. The second objective of this paper is therefore to investigate the usefulness of an extension of the FlowNet methodology with a machine learning proxy that can be used to produce predictions of H2S in the absence of a physics-based simulator module. The proxy is trained on historical liquid volume rates, seawater fractions, and H2S production data from a complex producing field, and then used to generate predictions of H2S production using FlowNet-based predictions of these same features as input.
We introduce the main characteristics of the field case, including a brief review of current understanding of reservoir souring taking place in the field. Several experiments are presented in which the source, type, and length of the training data time series are varied. Results indicate that, given a sufficient number of training data points, FlowNet is able to produce reliable predictions of conventional oil field quantities. The experiments performed with the machine learning proxy as an add-on, suggest that, at least for certain classes of production wells, useful predictions of H2S production can be obtained much faster and at much lower computational cost and complexity than would be possible with high-fidelity models. Finally, we discuss some current limitations and options to address them.
Despite their flexibility, the application of flow networks will be limited to physics that can be simulated with the available simulators. Prediction of phenomena with poorly understood underlying physics, chemistry, or, as in the case of reservoir souring, microbial ecology, may require a more data-driven approach. The second objective of this paper is therefore to investigate the usefulness of an extension of the FlowNet methodology with a machine learning proxy that can be used to produce predictions of H2S in the absence of a physics-based simulator module. The proxy is trained on historical liquid volume rates, seawater fractions, and H2S production data from a complex producing field, and then used to generate predictions of H2S production using FlowNet-based predictions of these same features as input.
We introduce the main characteristics of the field case, including a brief review of current understanding of reservoir souring taking place in the field. Several experiments are presented in which the source, type, and length of the training data time series are varied. Results indicate that, given a sufficient number of training data points, FlowNet is able to produce reliable predictions of conventional oil field quantities. The experiments performed with the machine learning proxy as an add-on, suggest that, at least for certain classes of production wells, useful predictions of H2S production can be obtained much faster and at much lower computational cost and complexity than would be possible with high-fidelity models. Finally, we discuss some current limitations and options to address them.
Session Chair
Ahmed Elsheikh
Professor
Heriot-Watt University
Session Co-Chair
Jeroen Vink
Shell Global Solutions Intl. B.V.
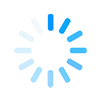