Uncertainty Quantification and Optimization - Data assimilation/History Matching/ CLM II
Tracks
Track 2
Monday, September 5, 2022 |
3:30 PM - 5:10 PM |
Room 1.2 |
Speaker
Ms Bettina Jenei
TU Clausthal
A novel application of Mahalanobis distance calculation in assisted history matching
3:30 PM - 3:55 PMSummary
The Mahalanobis distance is a statistical distance and is often used in various machine learning tasks to perform clustering analysis. This paper presents its application to history matching. This new extension, so-called rock typing coupled with the adjoint method, is proposed to maintain the geological consistency between model parameters of different rock types. The rock types have different porosity and permeability ranges, relative permeability curves, and different connate water and residual oil saturation.
In general, most gradient-based history matching tools honour the minimum and maximum geological constraints, but the link between the different rock type-dependent parameters may not be maintained. This leads to questionable geological admissibility of the entire model and, thus, calls for the necessity of removing or minimising inconsistencies.
This paper shows how the use of Mahalanobis distance calculation can suggest better and more plausible results. It presents the theory, applied methodology and the applicability of Mahalanobis distance calculation in a new history matching workflow that improves geological consistency, including the rock types. The rock type is changed with corresponding parameters at the grid-block level based on the porosity and horizontal permeability change with the rock typing history matching workflow. The so-called rock-typing extension of the history matching workflow allows parameters to be modified co-dependently according to the rock type definition based on the porosity and the permeability adjustments suggested by adjoint-based sensitivity calculations. The Mahalanobis distance is associated with the rock types through their porosity and permeability correlations. Therefore, it guides the correction step and determines the appropriate rock type based on the underlying statistical information.
History matching was performed on a simple synthetic model, a quarter of a five-spot pattern with the standard and the rock-typing extended workflow. Comparison results show significant improvements in history matching quality in terms of geological consistency with fewer iterations or within the same number of iterations but with favourable objective function values.
With the help of Mahalanobis distance, the novel approach has successfully preserved the geological consistency of the models during the history matching process.
In general, most gradient-based history matching tools honour the minimum and maximum geological constraints, but the link between the different rock type-dependent parameters may not be maintained. This leads to questionable geological admissibility of the entire model and, thus, calls for the necessity of removing or minimising inconsistencies.
This paper shows how the use of Mahalanobis distance calculation can suggest better and more plausible results. It presents the theory, applied methodology and the applicability of Mahalanobis distance calculation in a new history matching workflow that improves geological consistency, including the rock types. The rock type is changed with corresponding parameters at the grid-block level based on the porosity and horizontal permeability change with the rock typing history matching workflow. The so-called rock-typing extension of the history matching workflow allows parameters to be modified co-dependently according to the rock type definition based on the porosity and the permeability adjustments suggested by adjoint-based sensitivity calculations. The Mahalanobis distance is associated with the rock types through their porosity and permeability correlations. Therefore, it guides the correction step and determines the appropriate rock type based on the underlying statistical information.
History matching was performed on a simple synthetic model, a quarter of a five-spot pattern with the standard and the rock-typing extended workflow. Comparison results show significant improvements in history matching quality in terms of geological consistency with fewer iterations or within the same number of iterations but with favourable objective function values.
With the help of Mahalanobis distance, the novel approach has successfully preserved the geological consistency of the models during the history matching process.
Dr Xiaodong Luo
Norwegian Research Centre (NORCE)
Ensemble reservoir data assimilation with generic constraints
3:55 PM - 4:20 PMSummary
This work investigates an ensemble-based workflow to simultaneously handle generic (possibly nonlinear) equality and inequality constraints in reservoir data assimilation problems. The proposed workflow is built upon a recently proposed umbrella algorithm, called the generalized iterative ensemble smoother (GIES), and inherits the benefits of ensemble-based data assimilation algorithms in geoscience applications. Unlike the traditional ensemble assimilation algorithms, the proposed workflow admits cost functions beyond the form of nonlinear-least-squares, and has the potential to develop an infinite number of constrained assimilation algorithms. In the proposed workflow, we treat data assimilation with constraints as a constrained optimization problem. Instead of relying on a general-purpose numerical optimization algorithm to solve the constrained optimization problem, we derive an (approximate) closed form to iteratively update model variables, but without the need to explicitly linearize the (possibly nonlinear) constraint systems. The established model update formula bears similarities to that of an iterative ensemble smoother (IES). Therefore, in terms of theoretical analysis, it becomes relatively easy to transit from an ordinary IES to the proposed constrained assimilation algorithms, and in terms of practical implementation, it is also relatively straightforward to implement the proposed workflow for users who are familiar with the IES, or other conventional ensemble data assimilation algorithms like the ensemble Kalman filter (EnKF). Apart from the aforementioned features, we also develop efficient methods to handle two noticed issues that would be of practical importance for ensemble-based constrained assimilation algorithms. These issues include localization in the presence of constraints, and the (possible) high dimensionality induced by the constraint systems. We use one 2D and one 3D case studies to demonstrate the performance of the proposed workflow. In particular, the 3D example contains experiment settings close to those of real field case studies. In both case studies, the proposed workflow achieves better data assimilation performance in comparison to the choice of using an original IES algorithm. As such, the proposed workflow has the potential to further improve the efficacy of ensemble-based data assimilation in practical reservoir data assimilation problems.
Mr Mohammad Nezhadali
Phd Student
NORCE Norwegian Research Centre
Towards application of multilevel data assimilation in realistic reservoir history-matching problems
4:20 PM - 4:45 PMSummary
Harnessing sufficient computational resources is one of the main constraints in the domain of computational statistics. In ensemble-based data assimilation (DA), this constraint results in a limited ensemble size which in turn results in high sampling errors. In case of large amounts of simultaneous data, e.g. seismic data, these sampling errors manifest themselves in severe underestimation of uncertainties in posterior distributions. This problem is known as ensemble collapse. The traditional tool to mitigate this problem is localization. As the term implies, it annihilates spurious non-local correlations but does not allow for true non-local correlations. An alternative approach is use of lower-fidelity modeling which reduces the computational cost per realization and thus renders a larger ensemble size. However, it also entails larger numerical errors. A multilevel model is a set of models consisting hierarchies of both computational accuracy and cost. Accordingly, Multilevel Data Assimilation (MLDA) attempts to generate a better balance between the statistical and numerical errors by utilizing a multilevel model for the forecast step of the DA.
There have been several MLDA algorithms developed recently which have demonstrated promising results in assimilation of inverted seismic data in simplistic reservoir models. The multilevel attribute of these algorithms has been the coarseness of the spatial grid in the forward model. In this research, we examine one of these algorithms for assimilation of inverted seismic data in a more realistic petroleum reservoir problem. In doing so, a new method for coarsening the spatial grid is performed. This method allows for better inclusion of important geological features, such as faults. Additionally, certain adjustments are implemented so that the algorithm can handle storing and operating on large amounts of data efficiently. To assess the performance of the devised method, a numerical experiment is conducted, and the results obtained from this algorithm are compared with those of traditional DA methods.
There have been several MLDA algorithms developed recently which have demonstrated promising results in assimilation of inverted seismic data in simplistic reservoir models. The multilevel attribute of these algorithms has been the coarseness of the spatial grid in the forward model. In this research, we examine one of these algorithms for assimilation of inverted seismic data in a more realistic petroleum reservoir problem. In doing so, a new method for coarsening the spatial grid is performed. This method allows for better inclusion of important geological features, such as faults. Additionally, certain adjustments are implemented so that the algorithm can handle storing and operating on large amounts of data efficiently. To assess the performance of the devised method, a numerical experiment is conducted, and the results obtained from this algorithm are compared with those of traditional DA methods.
Mr Tarek Diaa-eldeen
Norwegian University of Science and Technology (NTNU)
System-Theoretic Ensemble Generation in Ensemble-Based History Matching
4:45 PM - 5:10 PMSummary
Reservoir model updating is an essential component in the closed-loop reservoir management and model-based production optimization. Ensemble-based methods, such as the ensemble Kalman filter (EnKF) and the ensemble smoother (ES), have been widely used as feasible alternatives that extend the application of the standard Kalman filtering techniques to such high-dimensional systems with inherent nonlinearities. However, the performance of the ensemble-based algorithms highly depends on the number and the distribution of the initial samples. In the case of linear dynamics, for instance, the analysis state vector is searched for in the subspace spanned by the initial state vectors. Therefore, ensemble initiation is essential to the performance of ensemble-based data assimilation approaches. In this paper, a system-theoretic method based on the observability characteristics of the underlying system is introduced to generate the initial ensemble realizations in ensemble-based history matching. Firstly, a generic approach using algorithmic differentiation is derived to obtain the linearized model of the reservoir with respect to both the dynamic (state) and static (parameter) variables directly from the numerical simulator. Then, the system's observability is analyzed, and an ensemble-based history matching is initiated by perturbing an initial guess in the directions of the high-observable vectors in the reservoir, instead of the traditional random perturbation. This, in addition, guarantees the orthogonality of the generated perturbations, and consequently, reduces the redundancy within the realizations. The statistical properties of the generated ensemble are analyzed, and the overall performance of the algorithm is assessed on the basis of a history matching twin experiment of a two-phase synthetic reservoir, and compared with the performance of the random sampling strategy. The ensemble randomized maximum likelihood algorithm (EnRML) is used as the assimilation algorithm in this study; however, the method is also applicable to the other ensemble-based assimilation algorithms. Numerical experiments show promising results for the proposed observability-based sampling strategy over the random sampling strategy in terms of the prediction errors of estimating the directional permeability field of subsurface porous media from noisy sparse production data.
Session Chair
Louis Durlofsky
Stanford University
Session Co-Chair
Mo Sayyafzadeh
Senior Research Scientist
CSIRO
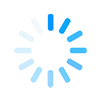